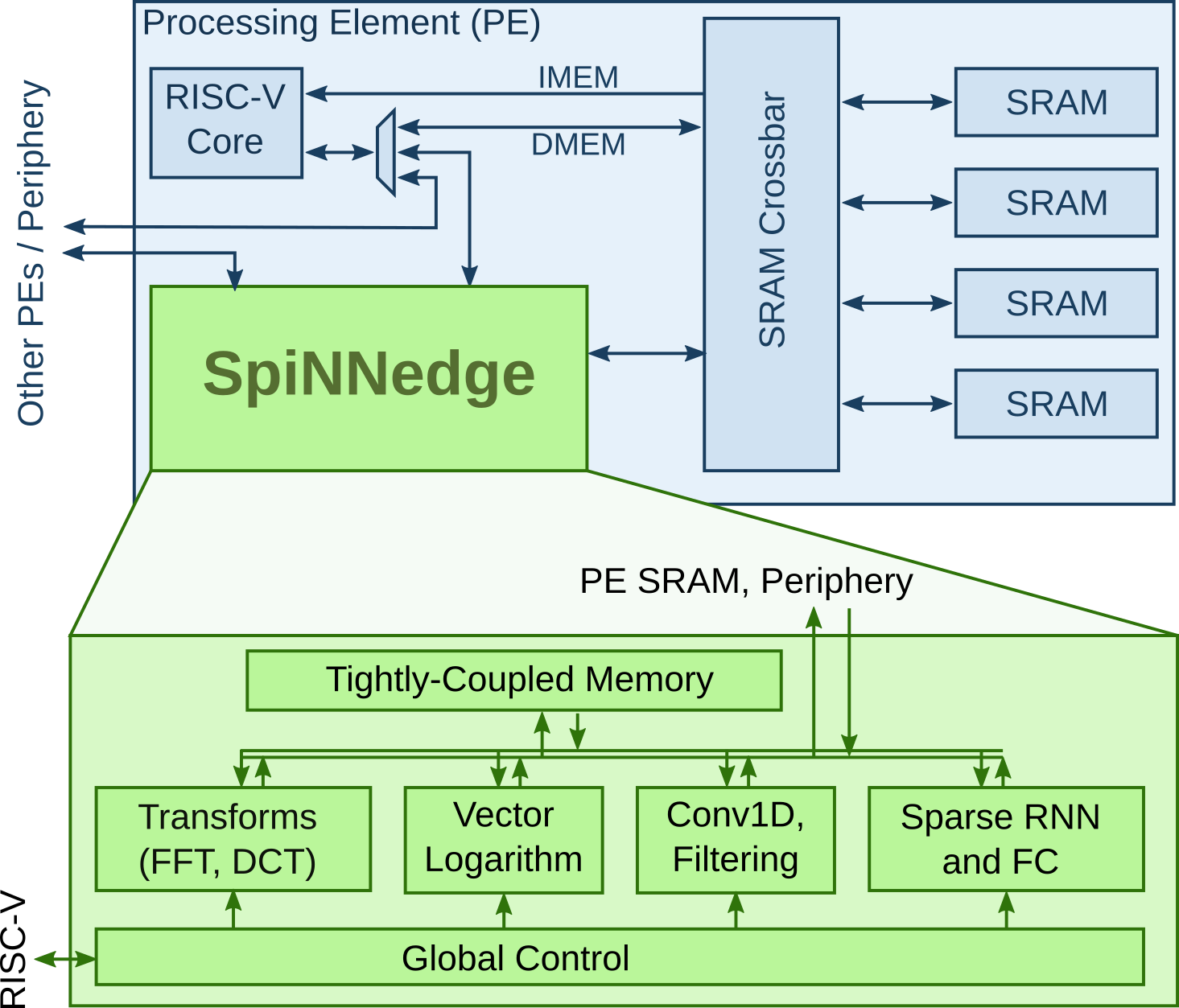
The SpiNNedge accelerator is designed for ultra-low-power processing and classification of time series from sensors in real time. It combines DSP functions for data preprocessing and feature extraction (frequency transforms, windowing, filtering, logarithm) with classification based on recurrent neural networks (RNN). The RNN module exploits sparsity for significantly reduced storage and processing effort. The accelerator performs individual processing layers autonomously. Complex layers can be flexibly assembled from base operations via a global control module. The SpiNNedge accelerator is specifically optimized for audio processing tasks like keyword spotting, e.g. supporting MFCC feature extraction from raw audio data. A backend for the TVM compiler framework, including RISC-V code generation, quantization and data handling, allows for easy deployment of RNN models onto the accelerator. Due to the shared memory between RISC-V and SpiNNedge, mixed deployment schemes can be realized with virtually no overhead, making the architecture adaptable to new user requirements even after manufacturing.
The SpiNNedge accelerator has been finalized in a first version and verified via an FPGA prototype and a test chip. Commercial availability is planned to be realized via the TU Dresden start-up SpiNNcloud Systems GmbH.
TU Dresden
Johannes Partzsch
Mommsenstr. 12
01069 Dresden
Deutschland